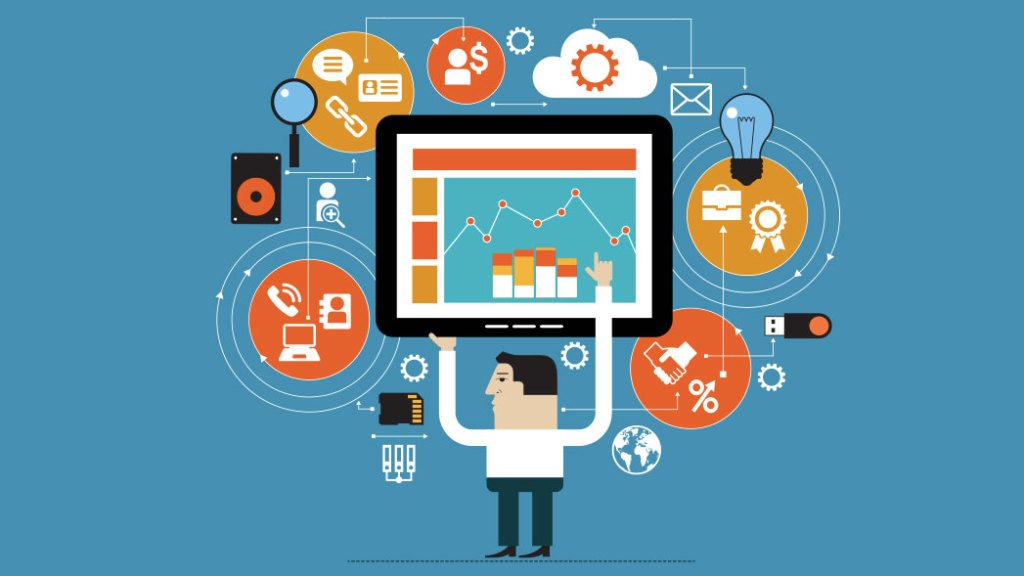
Generative AI for Genomic Data Analysis: Advancing Personalized Treatments
The rapid advancements in artificial intelligence (AI) have opened new doors in the realm of healthcare, particularly in the field of genomics. By analyzing vast amounts of genetic data, Generative AI for genomic data analysis is transforming how healthcare providers create personalized treatments. The integration of AI in genomic research is revolutionizing the approach to personalized medicine, allowing for more targeted and effective treatment strategies tailored to individual patients’ genetic profiles.
Generative AI in Genomics: What is it?
Genomics and AI represent the confluence of two powerful technologies. Genomic data refers to an individual’s complete set of DNA, which contains valuable information about their susceptibility to certain diseases, how they may respond to drugs, and their overall health profile. However, the sheer volume of data contained within an individual’s genome is immense, making it difficult to analyze manually. This is where machine learning in genomics comes into play, and more specifically, generative AI.
Generative AI uses advanced algorithms to create new data patterns based on the existing genomic information. These AI-driven models can predict how certain genetic variations will affect disease progression or treatment response. This is crucial for personalized medicine, as understanding the specific genetic factors of each patient enables healthcare providers to design treatment plans tailored to their unique needs.
The Role of Generative AI in Genomic Data Analysis
Generative AI for genomic data analysis is a critical tool for advancing personalized treatments. One of the most significant ways this technology is used is in the analysis of massive genomic datasets. The human genome consists of approximately 3 billion base pairs, and analyzing this data requires computational power that only AI systems can provide. By processing this data efficiently, AI algorithms can identify mutations, genetic markers, and patterns that are associated with specific health outcomes.
- Predicting Disease Risk: One of the primary applications of AI-driven healthcare data is predicting an individual’s risk for developing certain diseases. For example, by analyzing a patient’s genetic data, AI can identify predispositions to diseases such as cancer, Alzheimer’s, or diabetes. This allows healthcare providers to implement early interventions or preventive treatments that are tailored to the individual’s genetic profile.
- Drug Response Prediction: Not all patients respond to medications in the same way, and personalized medicine with AI enables healthcare professionals to predict how a patient will react to specific treatments. This is particularly beneficial for patients with chronic conditions or those undergoing cancer treatment, where precision medicine can make a significant difference in outcomes.
- Gene Editing and Therapies: Another exciting area where machine learning in genomics is making strides is in gene therapy. AI can assist in identifying which genes need to be targeted for editing or therapy to treat genetic disorders, enabling more effective treatments for diseases that were previously difficult to manage.
Personalized Medicine with AI: Precision Healthcare for the Future
Personalized medicine with AI represents a shift from the traditional one-size-fits-all approach to healthcare. By using healthcare AI for DNA analysis, treatments are no longer based solely on general population data but are instead customized based on each individual’s genetic makeup. This shift towards personalized medicine ensures that treatments are more effective, reducing the trial-and-error period often associated with finding the right medications or therapies.
A 2021 study published in Nature Medicine found that AI-based genomic analysis led to a 30% increase in the accuracy of predicting drug response in cancer patients (Nature Medicine, 2021). This demonstrates the potential for AI-driven healthcare data to improve outcomes significantly, particularly in complex diseases where traditional methods may fall short.
- Improved Accuracy in Diagnosis: AI models can detect even the smallest genetic mutations that may go unnoticed by traditional methods, leading to more accurate diagnoses and better patient outcomes.
- Faster Treatment Development: AI systems can process vast amounts of genomic data quickly, speeding up the development of personalized treatments and new drugs.
AI in Healthcare: The Role of Generative AI in DNA Analysis
The role of healthcare AI for DNA analysis is becoming increasingly prominent in precision medicine. AI systems can scan an individual’s DNA to identify patterns that correlate with certain diseases, treatments, or health conditions. This analysis can then be used to predict how a disease will progress or how a patient will respond to treatment, enabling more personalized care.
For instance, in cancer treatment, Genomics and AI can work together to identify genetic mutations that drive cancer growth. AI can then recommend treatments that target these specific mutations, leading to more effective and less toxic therapies. This approach, known as precision oncology, is one of the most promising areas of AI application in healthcare today.
Machine Learning in Genomics: The Backbone of AI-Driven Healthcare
Machine learning in genomics is the foundation upon which generative AI operates. By learning from large datasets, machine learning models can identify patterns and make predictions that are critical for advancing genomic medicine. This includes everything from identifying disease-associated genetic markers to predicting treatment outcomes.
One of the key benefits of AI-driven healthcare data is that it continues to improve over time. As more data is collected and analyzed, the algorithms become more accurate in their predictions, leading to better patient outcomes. This continuous learning process is particularly valuable in the ever-evolving field of genomics, where new discoveries are made regularly.
- Continuous Learning: AI systems improve their predictive accuracy as more genomic data becomes available, making them increasingly effective in personalizing treatment plans.
- Enhanced Data Interpretation: By processing complex genomic datasets, machine learning models can uncover insights that are difficult or impossible for humans to detect.
Addressing Bias in AI for Genomic Data
While the potential for AI in genomics is vast, it is essential to address the issue of bias in AI. If AI models are trained on datasets that are not diverse, the resulting predictions may be skewed. This can lead to disparities in healthcare outcomes, particularly for underrepresented groups. Ensuring that AI systems are trained on diverse and representative datasets is crucial for creating equitable healthcare solutions.
A 2020 study found that AI models trained primarily on data from European populations were less effective at predicting disease risk in individuals of African descent (Cell, 2020). This highlights the importance of addressing bias in AI to ensure that the benefits of AI-driven genomic analysis are available to all patients, regardless of their background.
Emotion Recognition Technology in Genomic Data Analysis
While Emotion Recognition Technology is primarily used in areas like mental health and customer service, its potential applications in healthcare, including genomics, are beginning to emerge. By integrating emotion recognition tools into genomic analysis platforms, healthcare providers can offer a more holistic approach to patient care. For example, patients dealing with emotionally taxing genetic conditions may benefit from technologies that monitor their emotional states and adjust their treatment plans accordingly.
FAQs about Generative AI for Genomic Data Analysis
Q: How does generative AI help in genomic data analysis?
Generative AI helps by analyzing large volumes of genomic data to identify patterns, predict disease risk, and recommend personalized treatments based on an individual’s genetic makeup.
Q: Can AI predict how I will respond to a certain treatment?
Yes, personalized medicine with AI enables healthcare providers to predict how patients will respond to treatments by analyzing their genetic data. This allows for more targeted therapies and reduces the likelihood of adverse reactions.
Q: What are the risks of bias in AI for genomic data analysis?
Bias in AI can lead to inaccurate predictions, especially for underrepresented populations. Ensuring diverse datasets and regular auditing of AI models is essential to reducing these risks and creating equitable healthcare solutions.
Q: How is machine learning used in genomics?
Machine learning in genomics is used to identify genetic markers associated with diseases, predict disease progression, and develop personalized treatment plans. It continuously learns from new data, improving its predictive capabilities over time.
User Experience with AI-Driven Genomic Data Analysis
For patients, the use of AI-driven healthcare data in genomic analysis has been transformative. Patients who previously struggled to find effective treatments due to their unique genetic profiles now have access to personalized care that is tailored to their needs. For example, patients with rare genetic disorders are benefiting from AI’s ability to identify mutations that may have been missed by traditional methods.
Healthcare providers also report significant improvements in their ability to offer precise, effective treatments. The use of generative AI for genomic data analysis has allowed them to streamline the diagnostic process, reduce unnecessary treatments, and offer more effective therapies. In many cases, the application of AI has shortened the time it takes to reach a diagnosis, improving patient outcomes and reducing healthcare costs.
Conclusion
Generative AI for genomic data analysis is revolutionizing the field of personalized medicine by enabling healthcare providers to analyze vast amounts of genetic data quickly and accurately. By using Genomics and AI, healthcare professionals can predict disease risks, personalize treatment plans, and even aid in drug discovery. However, it is critical to address challenges like bias in AI to ensure that all patients benefit from these advancements equally.
As the field of AI-driven healthcare data continues to grow, the integration of technologies like Emotion Recognition Technology and Generative AI in Healthcare will further enhance the ability to provide personalized, holistic care. By embracing these technologies, the future of healthcare promises to be more precise, efficient, and inclusive than ever before.